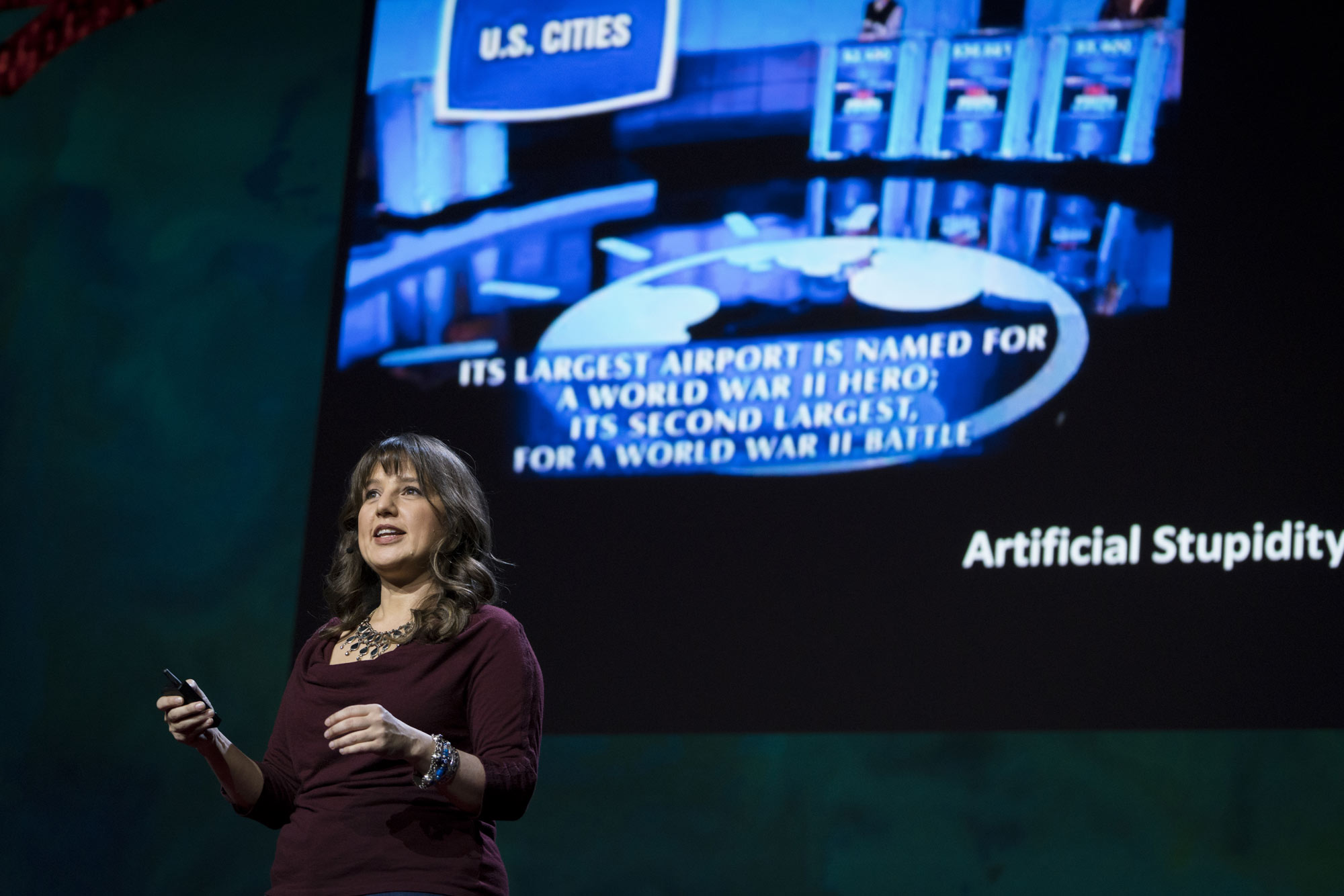
Zeynep Tufekci looks at our growing use of machine learning in everyday tasks — and asks us to examine the hidden bias (and even artificial stupidity) that AI may bring in its wake. Photo by Bret Hartman/TED.
Who would have thought when you left those high school math problems behind that you would one day be encountering algorithms on a daily basis? Zeynep Tufekci might have guessed; now an assistant professor at the University of North Carolina’s School of Information, Tufekci’s first job as a teenager was as a computer programmer. So it’s no surprise that she is way more adept at decrypting the confusing and often misleading worlds of social media than most of us.
And while she’s perfectly comfortable to hold forth on exactly Facebook’s algorithm does, what troubles her more are the next generation of algorithms even more powerful and ominous. “There are computational systems that can suss out emotional states — even lying — from processing human faces, “ she says. “They are developing cars that could decide who to run over.”
If that sounds a bit dramatic, Tufekci is quick to caution that most machine learning systems won’t crash through walls like killer cars, but be invited in, like friends who can solve problems.
“We are now asking questions of computation that have no single right answers, like, ‘Who should our company hire?’ or ‘Which convict is more likely to re-offend?’ But we have no benchmarks for how to make decisions about messy human affairs.”
Still, that hasn’t stopped software companies from trying, fine-tuning and turbo-charging algorithms to take more and more data into account to deliver more and more answers. In traditional programming, a system is given a series of static commands and computes the answer. Modern algorithms are driven by so-called machine learning, an approach to computing that evolved from pattern recognition and prediction software. With machine learning, a system calculates its results by churning through and “learning from” loads of data — but how those results are arrived at could well be a mystery even to those who defined the task parameters.
“In the past decade, complex algorithms have made great strides,” says Tufekci. “They can recognize human faces. they can decipher handwriting. The downside is we don’t really understand what the system learned. In fact, that’s its power.”
The idea of a company or college using an advanced algorithm to sort through mountains of job or school applicants is exactly the kind of thing that worries the Turkish-born technosociologist. “Hiring in a gender- and race-blind way certainly sounds good to me,” she says. “But these computational systems can infer all sorts of things about you from your digital crumbs, even if you did not disclose these things.”
Among the inferences computers can make even without an explicit mention: sexual preference, political leaning, ethnic background, social class and more. “What safeguards do you have that your black box isn’t doing something shady? What if [your hiring algorithm] is weeding out women most likely to be pregnant in the next year? With machine learning, there is no variable labeled ‘higher risk of pregnancy.’ So not only do you not know what your system is selecting on, you don’t even know where to look to find out.”
Tufekci is not a Luddite, though — far from it. “Such a system may even be less biased than human managers,” she points out. “And it could well make good monetary sense as well. But is this the kind of society we want to build without even knowing we’ve done it? Because we’ve turned decision making over to machines we don’t totally understand?”
Machine learning doesn’t begin from a place of purposeful intention like traditional programming — it’s driven by data, which in and of itself can have a bias. One system that’s used by American courts in parole and sentencing decisions was scrutinized by ProPublica, which audited the algorithm. “They found it was wrongly labeling black defendants at twice the rate as white defendants.”
As another data point: When the story of the civil rights protests in Ferguson, Missouri, exploded in 2014, she saw it on her Twitter feed — which was not filtered algorithmically. But on the filtered Facebook? Not so much. “The story of Ferguson wasn’t algorithm friendly — it’s not Like-able,” she says. “Instead, that week Facebook’s algorithms highlighted the ALS ice-bucket challenge.” A wet t-shirt parade in the name of charity? Who wouldn’t click on that?
The decision is ours — but only if we can decide we want to make it. The more we surrender that choice, the more knowledge and power we surrender of the world around us.